Federated Learning as a Career Path: Navigating the Future of AI and Data Privacy
Introduction In the rapidly evolving world of artificial intelligence (AI) and machine learning (ML), Federated Learning (FL) emerges as a groundbreaking approach, balancing the need for robust data-driven models with privacy concerns. As businesses and organizations increasingly adopt FL, it opens up exciting career opportunities for aspiring AI professionals as presented in Figure 1.
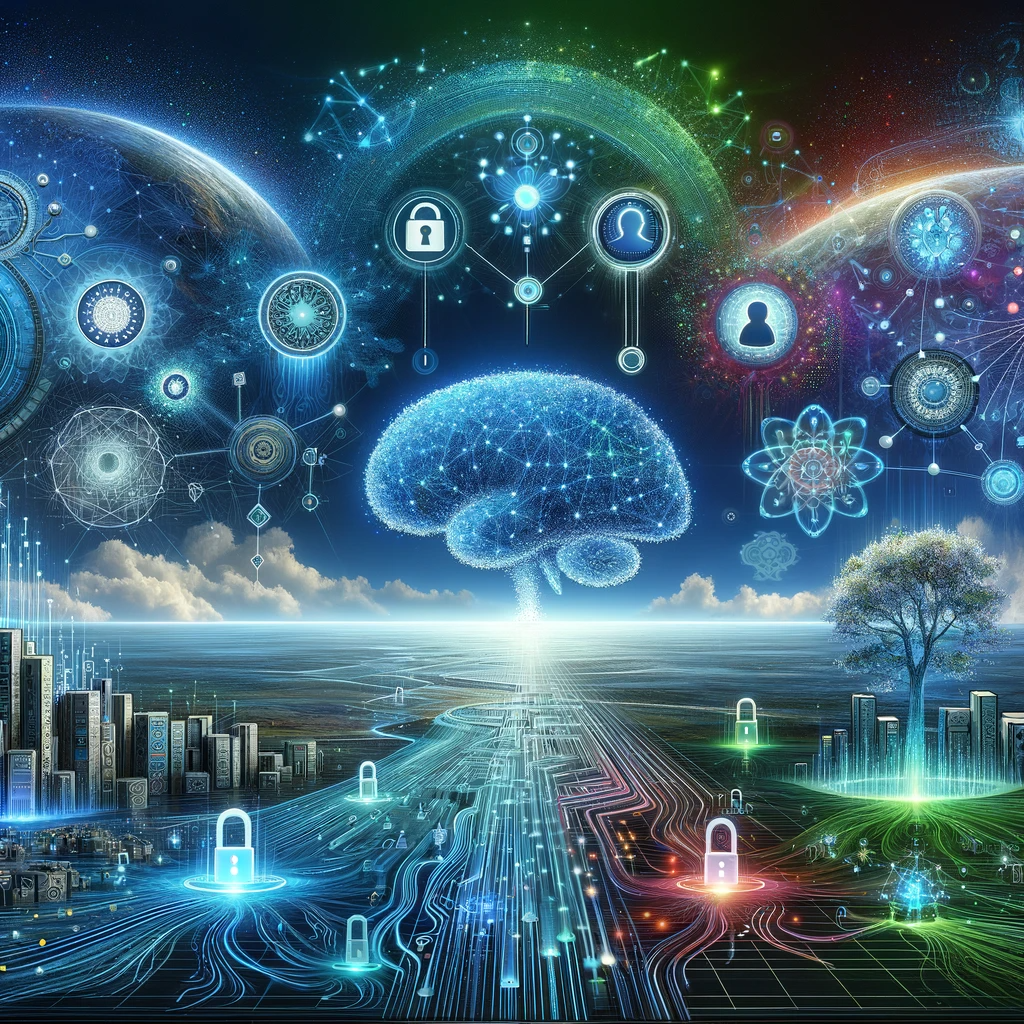
What is Federated Learning? Federated Learning is a decentralized approach to training ML models. Instead of pooling data in a central server, FL allows algorithms to be trained across multiple devices or servers holding local data samples. This method not only enhances privacy and security but also utilizes diverse data sources, making the models more robust and inclusive as depicted in Figure 2.
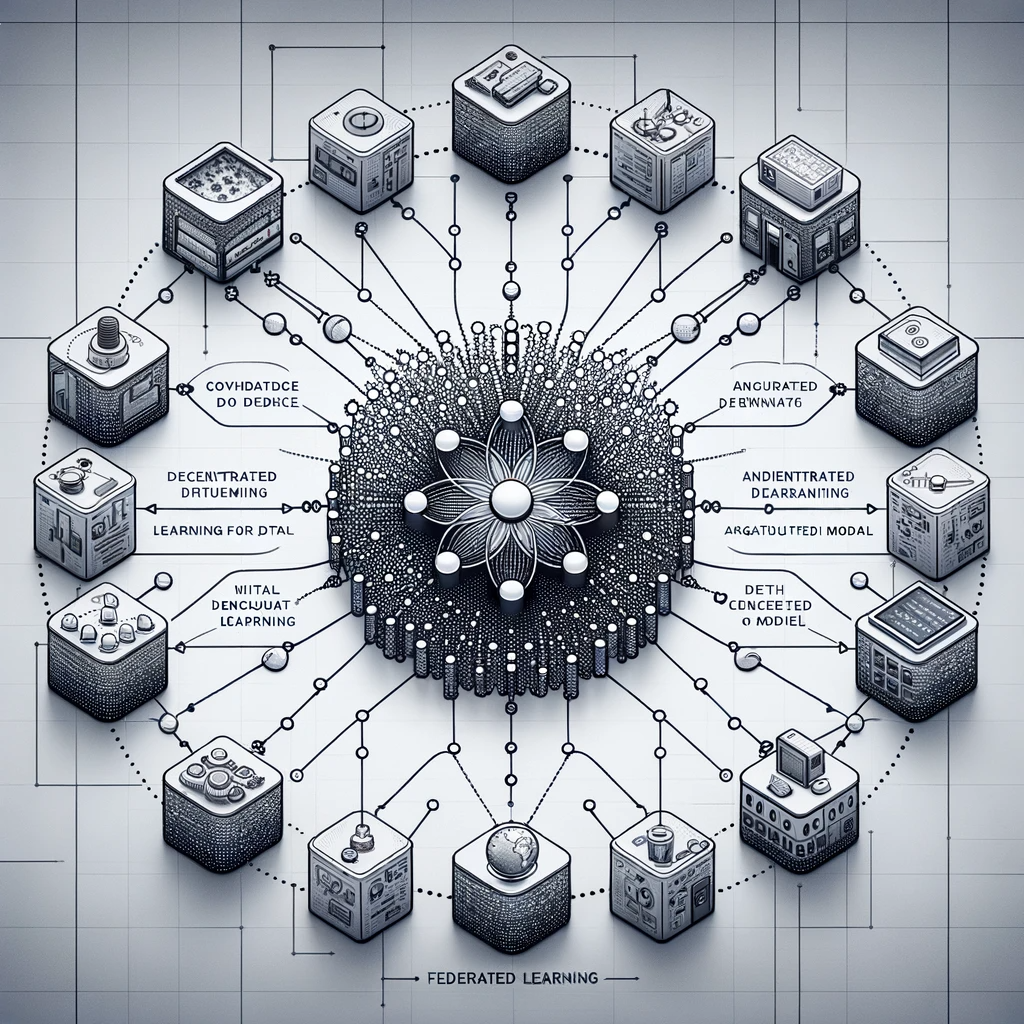
Why Choose a Career in Federated Learning?
- Growing Demand in Various Industries: Healthcare, finance, and telecommunications are just a few sectors where FL is becoming increasingly pivotal.
- Focus on Privacy: With rising concerns about data privacy, FL is gaining prominence for its ability to train models without compromising individual data.
- Interdisciplinary Opportunities: FL intersects with various domains, offering diverse career paths from engineering to ethical governance.
Figure 3 is crafted to showcase the increasing demand across various industries, the emphasis on privacy, and the diverse interdisciplinary opportunities.
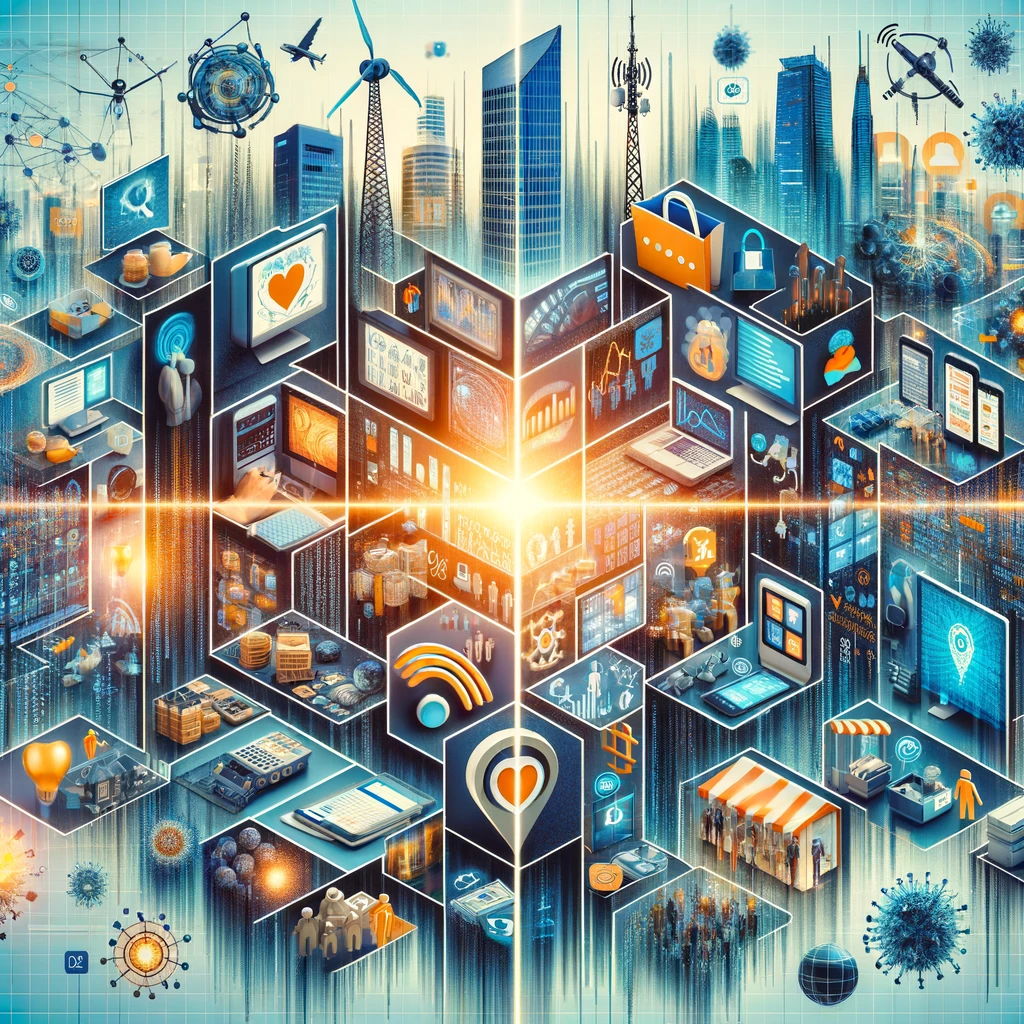
Skills Required for a Career in Federated Learning
- Machine Learning Expertise: Strong foundation in ML algorithms and data science.
- Programming Skills: Proficiency in languages like Python, along with knowledge of ML libraries.
- Understanding of Data Privacy: Knowledge of privacy-preserving techniques and regulations.
- Communication and Collaboration: Ability to work in interdisciplinary teams and communicate complex concepts effectively.
Figure 4 is presenting the key skills for a Federated Learning career encompass machine learning expertise, programming proficiency (especially in Python and ML libraries), data privacy knowledge, and effective communication and teamwork.
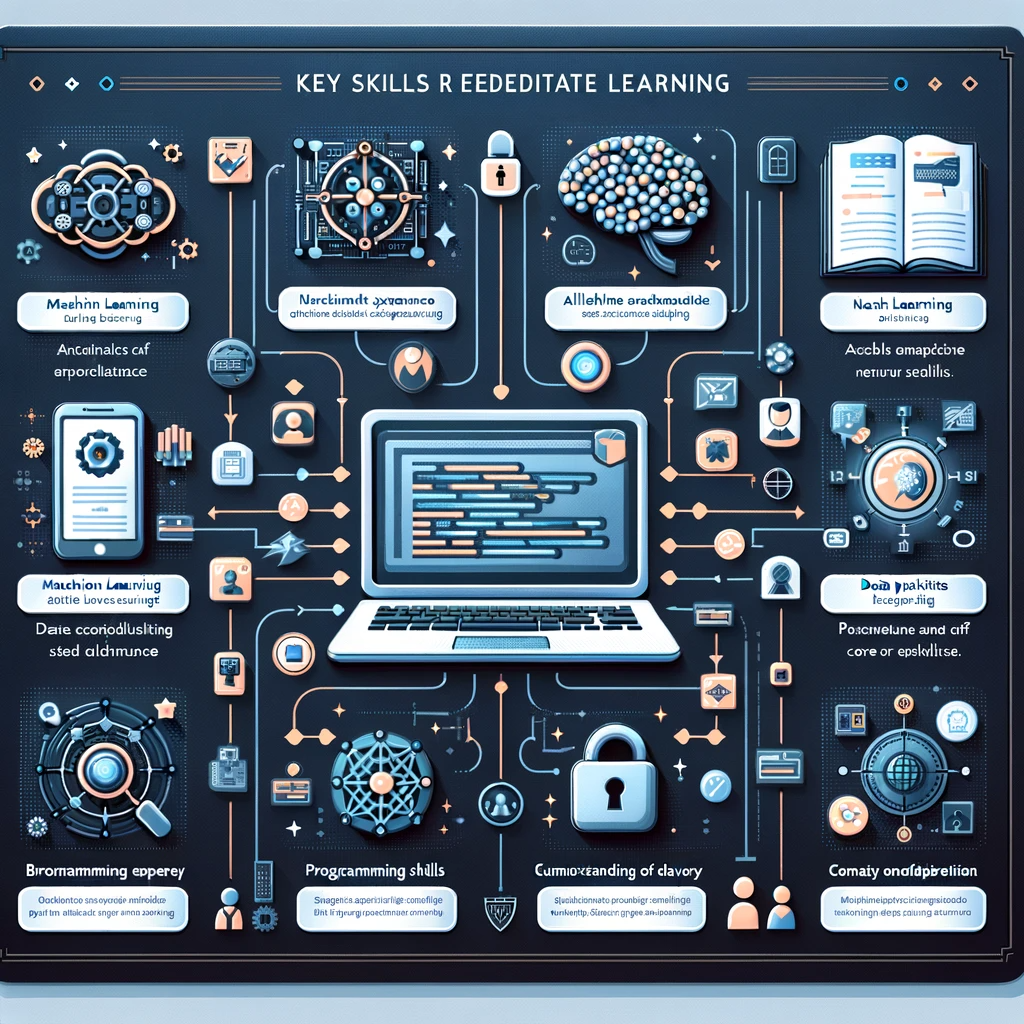
Educational Pathways To embark on a career in FL, one can start with a degree in computer science, data science, or a related field. Specialized courses and certifications in ML, data privacy, and Federated Learning are also beneficial as presented in Figure 5.
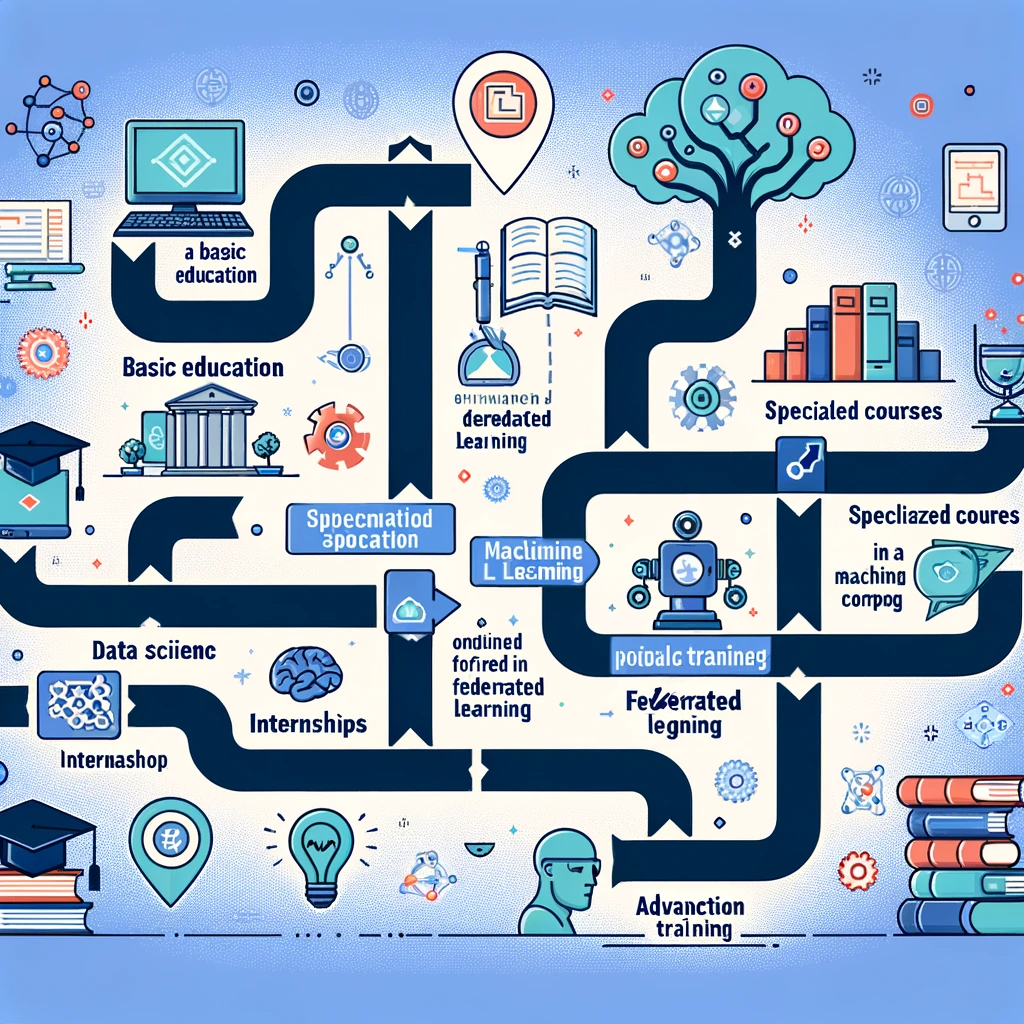
Career Opportunities and Roles
- Federated Learning Engineer: Developing and implementing FL algorithms.
- Data Privacy Analyst: Ensuring compliance with privacy regulations in FL models.
- Research Scientist: Innovating in the field of FL and contributing to academic research.
- Project Manager: Overseeing FL projects and ensuring collaboration across teams.
Federated Learning careers range from Engineers developing FL algorithms, Data Privacy Analysts ensuring regulatory compliance, Research Scientists driving innovation and research, to Project Managers overseeing projects and team collaboration as presented in Figure 6.
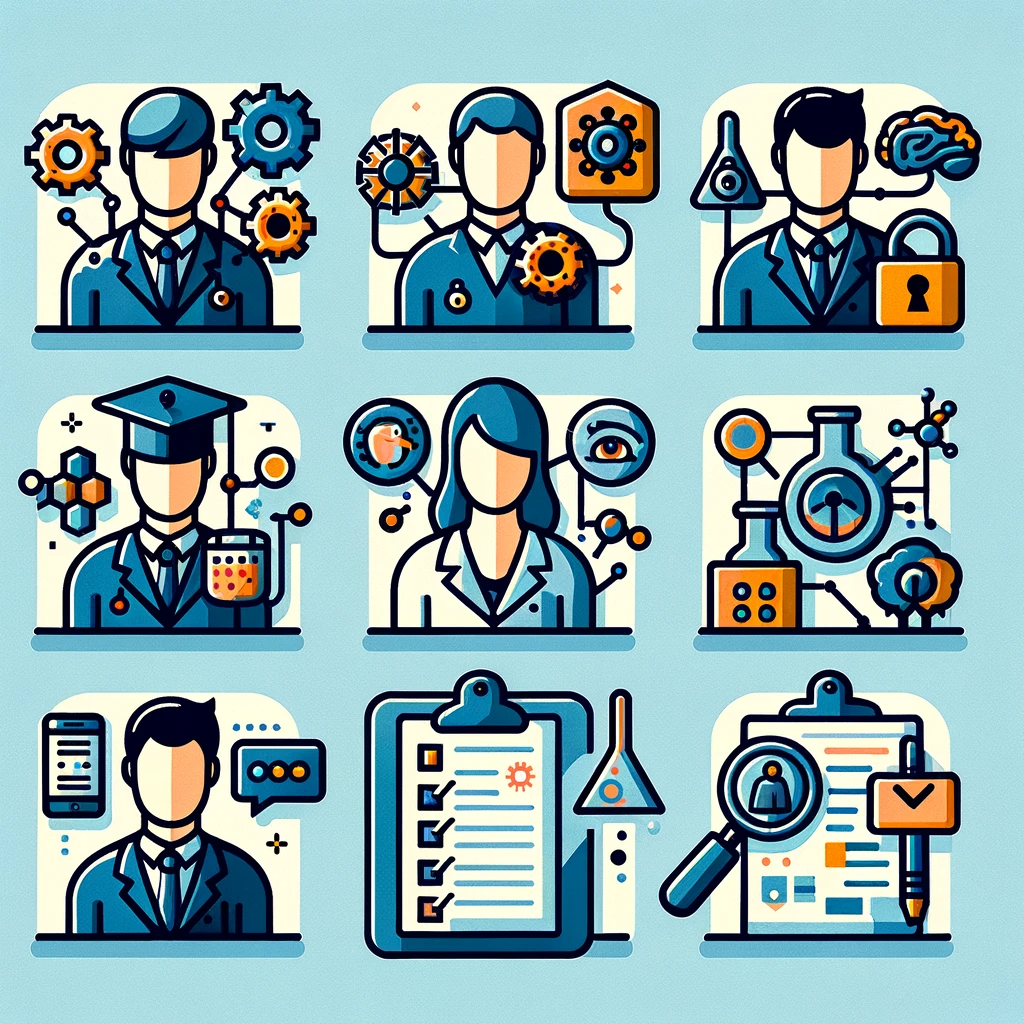
Challenges and Future Outlook While promising, FL is not without challenges, such as managing data heterogeneity and ensuring efficient communication protocols. However, the future is bright, with continuous advancements and increasing adoption across industries as presented in Figure 7.
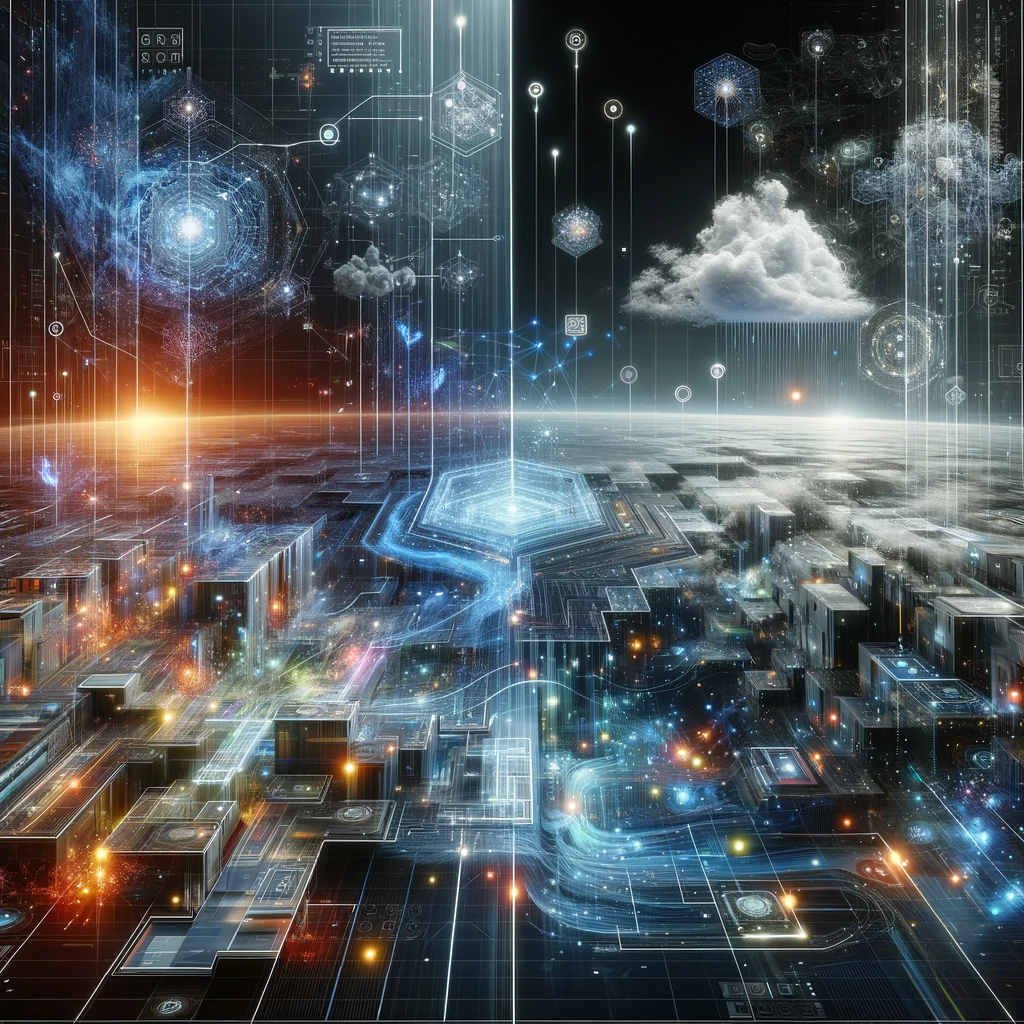
Conclusion Federated Learning stands at the forefront of a new era in AI, offering a promising career path for those interested in the intersection of technology, data privacy, and innovation. As industries continue to embrace FL, the demand for skilled professionals in this field is set to soar as presented in Figure 8.
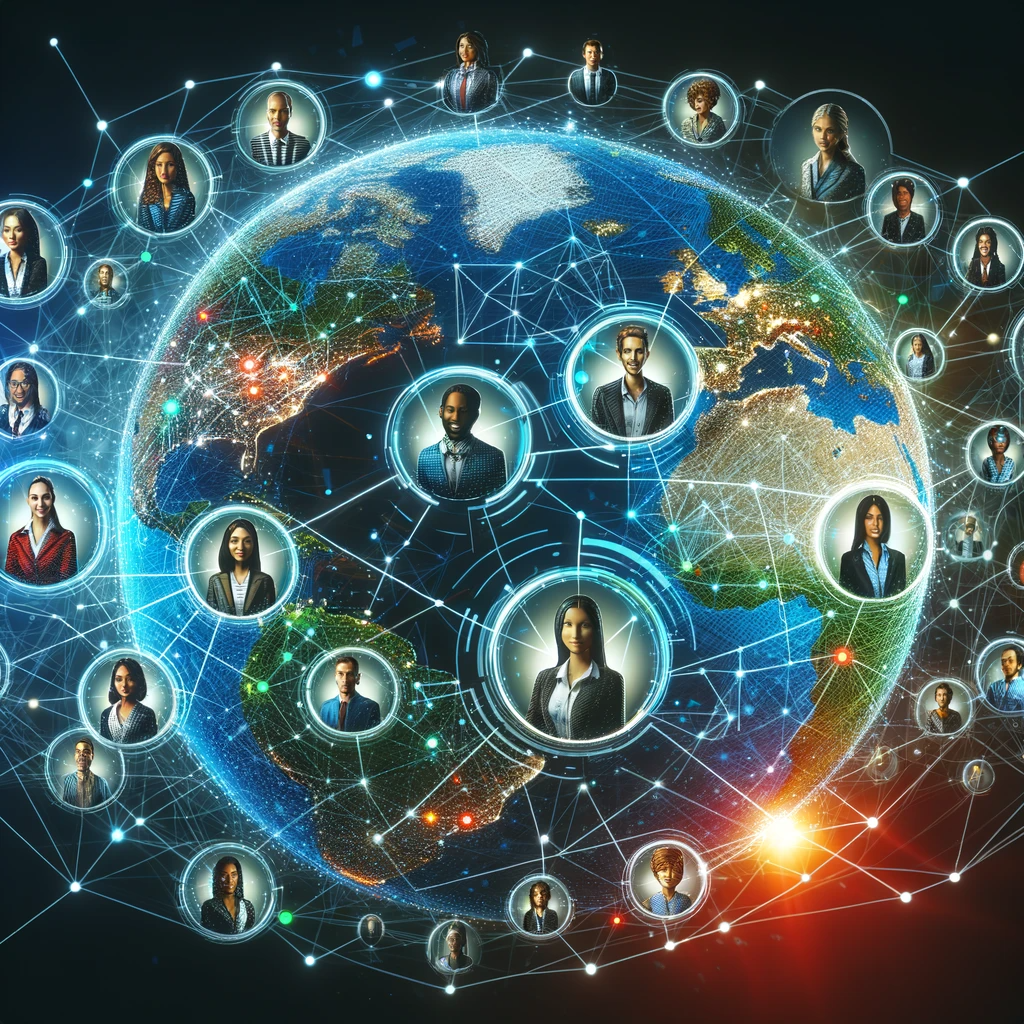